Agenda
Predictive Analytics World for Industry 4.0 2020
May 31-June 4, 2020
Click here to view the full 7-track agenda for the five co-located conferences at Machine Learning Week (PAW Business, PAW Financial, PAW Healthcare, PAW Industry 4.0, and Deep Learning World).
Pre-Conference Workshops - Sunday, May 31st, 2020
Full-day: 8:00am – 3:00pm
This one day workshop reviews major big data success stories that have transformed businesses and created new markets. Click workshop title above for the fully detailed description.
Full-day: 7:30am – 3:30pm
Gain experience driving R for predictive modeling across real examples and data sets. Survey the pertinent modeling packages. Click workshop title above for the fully detailed description.
Pre-Conference Workshops - Monday, June 1st, 2020
Full-day: 7:15am – 2:30pm
This one-day session surveys standard and advanced methods for predictive modeling (aka machine learning). Click workshop title above for the fully detailed description.
Full-day: 8:00am –3:00pm
Python leads as a top machine learning solution – thanks largely to its extensive battery of powerful open source machine learning libraries. It’s also one of the most important, powerful programming languages in general. Click workshop title above for the fully detailed description.
Full-day: 8:00am –3:00pm
Machine learning improves operations only when its predictive models are deployed, integrated and acted upon – that is, only when you operationalize it. Click workshop title above for the fully detailed description.
Predictive Analytics World for Industry 4.0 - Las Vegas - Day 1 - Tuesday, June 2nd, 2020
A veteran applying deep learning at the likes of Apple, Bosch, GE, Microsoft, Samsung, and Stanford, Mohammad Shokoohi-Yekta kicks off Machine Learning Week 2020 by addressing these Big Questions about deep learning and where it's headed:
- Late-breaking developments applying deep learning in retail, financial services, healthcare, IoT, and autonomous and semi-autonomous vehicles
- Why time series data is The New Big Data and how deep learning leverages this booming, fundamental source of data
- What's coming next and whether deep learning is destined to replace traditional machine learning methods and render them outdated
As principles purporting to guide the ethical development of Artificial Intelligence proliferate, there are questions on what they actually mean in practice. How are they interpreted? How are they applied? How can engineers and product managers be expected to grapple with questions that have puzzled philosophers since the dawn of civilization, like how to create more equitable and fair outcomes for everyone, and how to understand the impact on society of tools and technologies that haven't even been created yet. To help us understand how Google is wrestling with these questions and more, Jen Gennai, Head of Responsible Innovation at Google, will run through past, present and future learnings and challenges related to the creation and adoption of Google's AI Principles.
As the economy continues its uncertain path, businesses have to expand reliance on data to make sound decisions that directly impact the business - from managing cash flow to planning product promotion strategies, the use of data is at the heart of mitigating the risks of a recession as well as planning for a recovery. Predictive Analytics, powered by Artificial Intelligence (AI) & Machine Learning (ML), has always been at the forefront of using data for planning. Still, most companies struggle with the techniques, tools, and with lack of resources needed to develop and deploy predictive analytics in meaningful ways. Join dotData CEO, Ryohei Fujimaki to learn how automation can help Business Intelligence teams develop and add AI and ML-powered technologies to their BI stack through AutoML 2.0, and how organizations of all sizes can solve the predictive analytics challenge in just days without adding additional resources or expertise
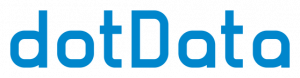
One of, if not THE, biggest impediments to Industrial firms realizing the efficiencies promised by "Industry 4.0" remains the access to quality, (near) real-time data. Ignoring the Purdue model, industrial firms should utilize cutting-edge cyber security tools to connect critical assets directly with professionals having the skills necessary to deploy advanced analytics solutions, optimizing machines and processes. Terry Miller, from Siemens, will evaluate a case study utilizing this architecture to capture and predict valve "stiction" in a Wastewater treatment plant flow loop.
TEMPA - TExt Mining with Predictive Analytics, for Engineering - An approach that allows users to face any unplanned outage in any engineering asset. The asset could be a gas turbine, an aircraft engine, an MRI machine, a locomotive, or a wind turbine. These assets normally provide both descriptive (text) and measured (numerical) output as data - which, when combined properly, have the potential to provide highly actionable insights. TEMPA enables this. This talk would focus on proven methods to automatically extract events from both textual information and operational data, monetize these insights improving profit, as applied in General Electric.
The three most important analytic innovations I’ve seen in (35 years of) extracting useful information from data are: Ensemble models, Target Shuffling, and Awareness of Cognitive Biases. Ensembles are competing models that combine to (very often) be more accurate than the best of their components. They seem to defy the Occam’s Razor tradeoff between complexity and accuracy, yet have led to a new understanding of simplicity. Target Shuffling is a resampling method that corrects for “p-hacking” or the “vast search effect” where spurious correlations are uncovered by modern methods’ ability to try millions of hypotheses. Target shuffling reveals the true significance of a model, accurately assessing its out-of-sample precision. Lastly, the increased understanding of our Cognitive Biases, and how deeply flawed our reasoning can be, reveals how projects can be doomed unless we seek out — and heed — constructive critique from outside.
Ari Kaplan will talk about his real-life Moneyball experiences of disruption in Major League Baseball front offices - and how artificial intelligence will disrupt every business industry. Having helped lead the adoption of data science throughout baseball, including creating the Chicago Cubs analytics department, he will lead lively discussion on how winning in baseball translates to winning in the finance industry, overcoming cultural resistance, and doing analytics at scale and velocity to win the race.

Data Science in general and Deep Learning in particular continue to reshape the future of the Energy sector across various segments. From exploration, development and production to downstream and new energies business, measurable value of digitalization has been observed in both efficiencies and savings. Deep Learning is one of the key underlying enablers for creating competitive advantage. This presentation provides an overview of some of use case applications and lessons learned from establishing a platform that progress ideas to embedded business enablers.
It is a rare opportunity for us to live in a time in which important new fields as Big Data Analytics (BDA) and the Internet of Things (IoT) are being born, maturing, and working together to advance technological progress. The following presentation will outline new opportunities and challenges for predictive analytics when applied to the field of Industrial IoT. It will also discuss various drivers and inhibitors that need to be considered, as well as successful strategies for offering predictive analytics for IoT.
Predictive Analytics World for Industry 4.0 - Las Vegas - Day 2 - Wednesday, June 3rd, 2020
Drawing from his experience as the chief data and analytics officer at three different companies, A. Charles Thomas – now chief data and analytics officer at General Motors – will share insights and lessons learned from both sides of the unique, two-pronged role he plays at GM.
First, Charles' team leverages analytics to enhance GM's traditional businesses, such as selling vehicles, OnStar, Warranty, SiriusXM, and others. The team generates insights to drive billion-dollar improvements in functions such as manufacturing, HR, Marketing, and Digital.
Second, Charles' team also drives revenue from their unique access to tremendous quantities of vehicle data. This includes direct licensing of connected vehicle data (e.g. GPS data to traffic and parking apps, media, retail, and insurance companies), as well as using these data to create new businesses in insurance, fleet management, and others.
In this keynote address to both the PAW Business and PAW Industry 4.0 audiences, Charles will share his unique insider's vantage.
This presentation is about making machine learning models and their decisions interpretable, and why that’s important and valuable
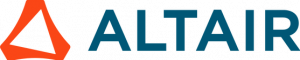
There has been an exponential increase in the number of electronic sensors, which now are driving every industry - right from Manufacturing to Aerospace. These sensors are capable of producing a lot of data. However, the sensors are known to be notorious for their jitters. These jitters often result in false alarms which could be troublesome for industrialists. In the session, we will take a deep dive into the state of the art approach. Auto-Encoders are a great product of Deep Learning Neural Networks, if used correctly they have the potential to save lot of cost on the asset.
Advances in additive manufacturing (AM) has allowed metal components to be fabricated quicker, and more cost-effectively than traditional metallurgical processes. For laser powder bed fusion (LPBF) AM, the area beneath the laser turns gaseous (vapor depression) while the area immediately surrounding turns to a liquid (melt pool). Once solidified they form the material microstructure, which determines the performance, lifespan, and physical features of the build. With in-situ data collected by ultrafast x-ray imaging, machine learning can be used to predict these geometries. Additionally, recommendations can be made for the underlying process parameters to determine optimal settings for future build characteristics.
What works in production is the only technology criteria that matters. Companies with successful high scale production IoT analytics programs like Philips, Anritsu, and Optimal+ show remarkable architectural similarities. The needs of IoT at production scale often transcend use case. Drill into some successful implementations in different industries, to study architectural structures that work, and why.
Learn to:
- Judge IoT data architecture choices critically and objectively
- Avoid traps that have cost other companies time and money and caused implementation failures
- Ensure AI and ML projects make it into production where they have real business impact
Organizations are routinely faced with the challenge of how to analyze their IoT data. This talk will focus on companies who collect data from their factory operations and are interested in predicting mechanical failures. The audience will get an overview of the entire process starting with how to formulate their business problem, perform feature engineering and build a predictive maintenance model using Python using both tradition/Deep learning techniques.
Research driven companies have a long ranging, unique and amazing data record. A constant challenge is to find relevant data for development scientists and customers to help them solving production problems. Typical approaches span from enterprise scale data lakes to sophisticated numerical simulations. This talk gives insights into use cases that transform a long established manufacturing company into a data-driven supplier.
Post-Conference Workshops - Thursday, June 4th, 2020
Full-day: 7:15am – 2:30pm
This one-day session reveals the subtle mistakes analytics practitioners often make when facing a new challenge (the “deadly dozen”), and clearly explains the advanced methods seasoned experts use to avoid those pitfalls and build accurate and reliable models. Click workshop title above for the fully detailed description.
Full-day: 8:00am –3:00pm
Gain the power to extract signals from big data on your own, without relying on data engineers and Hadoop specialists. Click workshop title above for the fully detailed description.
Full-day: 8:00am –3:00pm
This workshop dives into the key ensemble approaches, including Bagging, Random Forests, and Stochastic Gradient Boosting. Click workshop title above for the fully detailed description.
Full-day: 8:00am –3:00pm
During this workshop, you will gain hands-on experience deploying deep learning on Google’s TPUs (Tensor Processing Units) at this one-day workshop, scheduled the day immediately after the Deep Learning World and Predictive Analytics World two-day conferences. Click workshop title above for the fully detailed description.