Agenda
Predictive Analytics World for Financial Las Vegas 2019
June 16-20, 2019 – Caesars Palace, Las Vegas
This page shows the agenda for PAW Financial. Click here to view the full 7-track agenda for the five co-located conferences at Mega-PAW (PAW Business, PAW Financial, PAW Healthcare, PAW Industry 4.0, and Deep Learning World).
Session Levels:
Blue circle sessions are for All Levels
Red triangle sessions are Expert/Practitioner Level
Pre-Conference Workshops - Sunday, June 16th, 2019
Full-day: 8:30am – 4:30pm
This one day workshop reviews major big data success stories that have transformed businesses and created new markets. Click workshop title above for the fully detailed description.
Two and a half hour afternoon workshop:
This 2.5 hour workshop launches your tenure as a user of R, the well-known open-source platform for data analysis. Click workshop title above for the fully detailed description.
Pre-Conference Workshops - Monday, June 17th, 2019
Full-day: 8:30am – 4:30pm:
This one-day session surveys standard and advanced methods for predictive modeling (aka machine learning). Click workshop title above for the fully detailed description.
Full-day: 8:30am – 4:30pm:
Gain experience driving R for predictive modeling across real examples and data sets. Survey the pertinent modeling packages. Click workshop title above for the fully detailed description.
Full-day: 8:30am – 4:30pm:
This workshop dives into the key ensemble approaches, including Bagging, Random Forests, and Stochastic Gradient Boosting. Click workshop title above for the fully detailed description.
Full-day: 8:30am – 4:30pm:
This one-day introductory workshop dives deep. You will explore deep neural classification, LSTM time series analysis, convolutional image classification, advanced data clustering, bandit algorithms, and reinforcement learning. Click workshop title above for the fully detailed description.
Day 1 - Tuesday, June 18th, 2019
A veteran applying deep learning at the likes of Apple, Samsung, Bosch, GE, and Stanford, Mohammad Shokoohi-Yekta kicks off Mega-PAW 2019 by addressing these Big Questions about deep learning and where it's headed:
- Late-breaking developments applying deep learning in retail, financial services, healthcare, IoT, and autonomous and semi-autonomous vehicles
- Why time series data is The New Big Data and how deep learning leverages this booming, fundamental source of data
- What's coming next and whether deep learning is destined to replace traditional machine learning methods and render them outdated
In the United States, between 1500 and 3000 infants and children die due to abuse and neglect each year. Children age 0-3 years are at the greatest risk. The children who survive abuse, neglect and chronic adversity in early childhood often suffer a lifetime of well-documented physical, mental, educational, and social health problems. The cost of child maltreatment to American society is estimated at $124 - 585 billion annually.
A distinctive characteristic of the infants and young children most vulnerable to maltreatment is their lack of visibility to the professionals. Indeed, approximately half of infants and children who die from child maltreatment are not known to child protection agencies before their deaths occur.
Early detection and intervention may reduce the severity and frequency of outcomes associated with child maltreatment, including death.
In this talk, Dr. Daley will discuss the work of the nonprofit, Predict-Align-Prevent, which implements geospatial machine learning to predict the location of child maltreatment events, strategic planning to optimize the spatial allocation of prevention resources, and longitudinal measurements of population health and safety metrics to determine the effectiveness of prevention programming. Her goal is to discover the combination of prevention services, supports, and infrastructure that reliably prevents child abuse and neglect.
The research on the state of Big Data and Data Science can be truly alarming. According to a 2019 NewVantage survey, 77% of businesses report that "business adoption” of big data and AI initiatives are a challenge. A 2019 Gartner report showed that 80% of AI projects will “remain alchemy, run by wizards” through 2020. Gartner also said in 2018 that nearly 85% of big data projects fail. With all these reports of failure, how can a business truly gain insights from big data? How can you ensure your investment in data science and predictive analytics will yield a return? Join Dr. Ryohei Fujimaki, CEO and Founder of data science automation leader dotData, to see how Automation is set to change the world of data science and big data. In this keynote session, Dr. Fujimaki will discuss the impact of Artificial Intelligence and Machine Learning on the field of data science automation. Learn about the four pillars of data science automation: Acceleration, Democratization, Augmentation and Operationalization, and how you can leverage these to create impactful data science projects that yield results for your business units and provide measurable value from your data science investment.
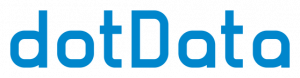
Understanding the intraday microstructure dynamics across different universes of stocks and markets is fundamental in designing any optimal trading strategy, especially for trading diverse portfolios of stocks. In this talk we discuss how modern machine learning techniques can be used in conjunction with dynamical modeling of the intraday phenomena to identify those trading strategies that move away from the general country/sector classifications but rather respect the stock's particular microstructure characteristics.
11:20 am - 11:40 am
This project sets forth the work to be performed for the Customer Acquisition Model, aiming to score the entire through-the-door (TTD) populations and eventually make optimal lending decisions. The work will be divided into two phases: Phase 1 will concentrate on building a Minimum Viable Product (MVP). This phase will leverage existing techniques used currently, where applicable, including the target, data sources and transformations. Phase 2 will conduct another round of data exploration with the purpose of identifying additional transformations to increase model lift over what was already achieved in Phase 1.
11:45 am - 12:05 pm
Understanding the problem to be solved is the most critical element in a successful project. A model that gets 99.3% accuracy to the wrong question does not help the client. And not being able to explain why the results occurred, particularly after a change, does not lead to success. It leads to frustration and the inability to use the model. Safety National's first Data Science Project is a clear example of having the right people in the process at the right time.
Financial institutions have long excelled at "analysis." We are overrun with reports, dashboards, key performance indicators and other metrics, and many financial organizations have a history of using this information to make data-driven decisions. But it is no longer enough. With rapid advances in technology and ever increasing stores of data, the opportunity is present for data scientists to dig in and get their hands dirty building real products and services for actual customers. It is time to trade information for engineering. This talk explores the shift from a consulting, insights-generation mindset for analytics to one of data-driven software development and what that means for financial institutions. How can you best structure these new hybrid analytics-engineering teams and how should you set them loose to generate value for the organization? Come to hear more.
Come and learn how predictive analytics can be combined to Decision Management and Notation (DMN) standard to provide business end users with a simple and clear depiction of their business decision context. Using a loan pre-qualification example we will demonstrate how DMN can leverage predictive analytics models captured in PMML. We will also show how the audit data generated from the DMN execution can be used for dashboarding, business intelligence, or fed back into the predictive model. This approach will enable you to clearly demonstrate to business users how predictive models directly contributes value into the day to day business operational decisions.
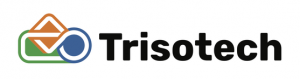
Many companies regardless of their size and years in business may not actually have an analytics team or may have a team of one. During my last speaking engagement at PAW I spoke with a great deal of folks who were interested in creating analytics units but didn't really know how to go about it or were under assumption that it would be very cost prohibitive. This is a case study of a team that consists of former spreadsheet guy, grad with a fresh masters in engineering, a former rocket scientist and two former workflow coordinators.
In this session, I will provide an overview of logistic regression, GLM logistic regression, decision tree, random forest, gradient boosting, neural networks, etc. Then, a comparison will be made through a case study about building a full life cycle of predictive model based on insurance datasets. Since the business goal through the feature engineering stages are similar given the same case study, the comparison of each method, its advantages and disadvantages, will include the feature selection, model building, model validation, and model testing stages. Also, model implementation and interpretability will be discussed and compared. Finally, we will discuss implementation of these methods in Python and R.
Wavelets have long been known for their strong ability in denoising and transforming signals, for example in speech recognition and image processing. More recently, Long Short Term Memory Networks (LSTMs) and Autoencoders started showing promising results in this space as well. Application is possible also to data sets generated by complex nonlinear processes with a low signal to noise ratio. Both Wavelets and LSTMs offer value through generating cleaner training data and ultimately driving deeper insights. In this session, we will show three concrete use cases: Analysis of Financial Time Series, Sales Forecasting and Credit Risk Analysis.

Day 2 - Wednesday, June 19th, 2019
Predictive modeling continues to play an important role in the claim process for Property & Casualty (P&C) insurers and Third Party Administrators (TPAs). This session focuses on the TPA environment utilizing the workers' compensation line of business as a case study into the rationale, implementation, and outcomes that follow the decision to deploy predictive modeling. The speaker will explain the environment of workers' compensation claims handling and the role of the TPA as it relates to assisting employers in managing risk. With this foundation, the session will move into how predictive modeling can be deployed in the claim process and specifically look at one TPAs efforts to increase efficiency and improve client outcomes. Participants of the session will walk away with the following information:
- Basics on the P&C TPA environment
- Opportunities for predictive modeling in the claim process
- Implementation challenges and pitfalls to consider regardless of industry
- Overcoming pitfalls
- Realizing outcomes in a dynamic environment
How can you predict portfolio delinquency? How do you consider hundreds of factors in assessing the risk in your portfolio, proactively? Bill Rachilla, Senior Product Manager for Loven System’s diwo solution, discusses the answers to these questions through use of a cognitive decision making platform.
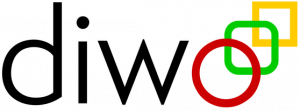
The use of AI in decision making processes brings efficiency and data-driven results, but also risks. Machine learning creates models which make predictions based upon patterns learned from past data. The reasoning behind these decisions is not available to the users of the models, or recipients dealing with the consequences of the decisions. E.g., a sales person doesn't know why a business is a good lead, and credit doesn't know why credit is denied. This is a case study on adding explanations to machine learning algorithms, so that users will have greater confidence and insight into machine-driven decisions.
Predictive models are increasingly used for important decisions such as which customers may open a financial account. These decisions affect the opportunities available to customers and drive business results. To maintain the customers' trust, it is important to be able to explain individual predictions. Likewise, it is important to explain the model logic for business managers, compliance professionals and regulators who expect fair decisions. This is not easy when using advanced techniques such as ensemble models. Mr. Duke will share Experian's recent advances in explainable AI technologies, with results in credit risk modeling, synthetic identity detection and fraud prevention.
In the insurance and banking industries, the track record of contributions made by women continues to grow. This is helping pave the way for future female scientists and analytics leaders. Predictive analytics and machine learning are no exception. At this panel session, learn from women in these fields what they've learned along the way, their wins and losses, and how they are helping others do the same. Our expert panelists will address questions such as:
- How can you best fit in and stand up as a woman in predictive analytics and machine learning?
- What are the key elements of being successful women scientists in these fields?
- What are the key elements of being successful women analytics leaders?
- How can you best build and manage your analytics team as a female analytics leader?
- How can you increase the count of women in your analytics team, especially in leadership roles?
- What are the differences from other science and engineering fields in terms of male domination?
- How do you suggest balancing work and personal life?
Data Science and machine learning practices have been well established in the financial industry for decades, vastly expanding the depth, breadth, and speed of analysis within securities trading and risk management, to name a few. Open source libraries and commercial automated machine learning platforms are evolving fast and being a “quant” is no longer a requirement to access and implement these advanced techniques. In this talk, we will review the evolution of tools in this space and highlight several use cases with broad application across the financial industry.
2:15 pm - 2:35 pm
"Build a better mousetrap and the world will beat a path to your door." Build a dozen mousetraps, with different triggers, bait, and alarms all meant for different species of mice, and the world will beat your door down. This presentation addresses the challenges of alert fatigue generated by successful predictive models. When your target audience is presented with multiple models recommending different and occasionally overlapping but important actions, there needs to be harmony in the message sent. This presentation examines the interactions of prioritization, frequency, severity development, consistency, messaging, and user socialization across multiple models for effective action.
2:40 pm - 3:00 pm
There have been ten US recessions since 1950. When is the next one? The answer matters because asset prices plunge in recessions, which creates both risk and opportunity. Forecasters answer this question by looking at leading economic indicators. We translate the thinking of forecasters into machine learning solutions. This talk explains the use of recurrent neural networks, which excel at learning historical patterns that don’t repeat, but rhyme. Our model anticipates the Great Recession from past data and exhibits lower error than established benchmarks. The proposed approach is broadly applicable to other prediction problems such as revenue and P&L forecasting.
3:30 pm - 3:50 pm
While many businesses understand the value of advanced analytics in decision making, operationalizing data science can be challenging. This session reveals how Enova International has been successful at integrating traditional operations with advanced analytics to turn fraud defense into a collaborative analytics function. Over time, through combining the latest technologies in data, machine learning and decision automation with manual investigations, Enova International has been able to attract and retain top analytics talent, mitigate fraud risk, improve profitability and deliver a better customer experience.
3:55 pm - 4:15 pm
Many businesses determine customer lifetime value (CLTV) in order to plan how to attract and retain customers. Traditionally, they use descriptive analytics to determine the average CLTV. However, with the expectation of receiving personalized services, these methods are inadequate. Predicting how long a new customer is expected to stay as a customer, and consequently their expected CLTV, companies can make decisions on the best way to serve them. In this talk, we will discuss practical tips and lessons learned in building machine learning models for determining CLTV including pitfalls to avoid and how deployment affects the model selection.
Predictive analytics has been a buzzword for a few years now. It has seen success for a wide range of applications. Within the Insurance industry, several applications have emerged, across underwriting, claims, marketing, and beyond. In this session, we will highlight examples of success factors that help sustain predictive analytics in a workers compensation insurance environment.
Post-Conference Workshops - Thursday, June 20th, 2019
Full-day: 8:30am – 4:30pm:
This one-day session reveals the subtle mistakes analytics practitioners often make when facing a new challenge (the “deadly dozen”), and clearly explains the advanced methods seasoned experts use to avoid those pitfalls and build accurate and reliable models. Click workshop title above for the fully detailed description.
Full-day: 8:30am – 4:30pm:
Gain the power to extract signals from big data on your own, without relying on data engineers and Hadoop specialists. Click workshop title above for the fully detailed description.
Full-day: 8:30am – 4:30pm:
During this workshop, you will gain hands-on experience deploying deep learning on Google’s TPUs (Tensor Processing Units) – held the day immediately after the Deep Learning World and Predictive Analytics World two-day conferences. Click workshop title above for the fully detailed description.