Agenda
Predictive Analytics World for Business 2020
May 31-June 4, 2020
Click here to view the full 7-track agenda for the five co-located conferences at Machine Learning Week (PAW Business, PAW Financial, PAW Healthcare, PAW Industry 4.0, and Deep Learning World).
TRACK TOPICS – The three tracks of the main two-day conference cover these topics:
Operationalization, management, best practices
Track 1
Machine learning methods & advanced topics
Track 2
Cross-industry business applications of machine learning
Track 3
TOPICS – The sessions across this two-day, three-track conference are grouped into the following four topics:
Operationalization, management, best practices
Track 1
Machine learning methods & advanced topics
Track 2
Cross-industry business applications of machine learning
Track 3
Session Levels:
Blue circle sessions are for All Levels
Red triangle sessions are Expert/Practitioner Level
Pre-Conference Workshops - Sunday, May 31st, 2020
Full-day: 8:00am – 3:00pm
This one day workshop reviews major big data success stories that have transformed businesses and created new markets. Click workshop title above for the fully detailed description.
Full-day: 7:30am – 3:30pm
Gain experience driving R for predictive modeling across real examples and data sets. Survey the pertinent modeling packages. Click workshop title above for the fully detailed description.
Pre-Conference Workshops - Monday, June 1st, 2020
Full-day: 7:15am – 2:30pm
This one-day session surveys standard and advanced methods for predictive modeling (aka machine learning). Click workshop title above for the fully detailed description.
Full-day: 8:00am –3:00pm
Python leads as a top machine learning solution – thanks largely to its extensive battery of powerful open source machine learning libraries. It’s also one of the most important, powerful programming languages in general. Click workshop title above for the fully detailed description.
Full-day: 8:00am –3:00pm
Machine learning improves operations only when its predictive models are deployed, integrated and acted upon – that is, only when you operationalize it. Click workshop title above for the fully detailed description.
Predictive Analytics World for Business - Las Vegas - Day 1 - Tuesday, June 2nd, 2020
A veteran applying deep learning at the likes of Apple, Bosch, GE, Microsoft, Samsung, and Stanford, Mohammad Shokoohi-Yekta kicks off Machine Learning Week 2020 by addressing these Big Questions about deep learning and where it's headed:
- Late-breaking developments applying deep learning in retail, financial services, healthcare, IoT, and autonomous and semi-autonomous vehicles-
- Why time series data is The New Big Data and how deep learning leverages this booming, fundamental source of data-
- What's coming next and whether deep learning is destined to replace traditional machine learning methods and render them outdated
As principles purporting to guide the ethical development of Artificial Intelligence proliferate, there are questions on what they actually mean in practice. How are they interpreted? How are they applied? How can engineers and product managers be expected to grapple with questions that have puzzled philosophers since the dawn of civilization, like how to create more equitable and fair outcomes for everyone, and how to understand the impact on society of tools and technologies that haven't even been created yet. To help us understand how Google is wrestling with these questions and more, Jen Gennai, Head of Responsible Innovation at Google, will run through past, present and future learnings and challenges related to the creation and adoption of Google's AI Principles.
As the economy continues its uncertain path, businesses have to expand reliance on data to make sound decisions that directly impact the business - from managing cash flow to planning product promotion strategies, the use of data is at the heart of mitigating the risks of a recession as well as planning for a recovery. Predictive Analytics, powered by Artificial Intelligence (AI) & Machine Learning (ML), has always been at the forefront of using data for planning. Still, most companies struggle with the techniques, tools, and with lack of resources needed to develop and deploy predictive analytics in meaningful ways. Join dotData CEO, Ryohei Fujimaki to learn how automation can help Business Intelligence teams develop and add AI and ML-powered technologies to their BI stack through AutoML 2.0, and how organizations of all sizes can solve the predictive analytics challenge in just days without adding additional resources or expertise.
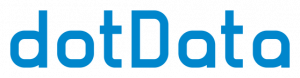
Track Co-Chair: James Taylor
9:30 am - 9:50 am
Digital decisioning operationalizes machine learning and artificial intelligence so your systems act intelligently on your behalf, making precise, consistent, real-time decisions at every touch point. Digital decisioning applies machine learning and artificial intelligence at scale to automate the decisions essential for more profitable, more customer-centric, and more digital business operations.
In this session, thought leader James Taylor will outline a proven approach to digital decisioning, by which large, established companies gain value from machine learning. He'll cover examples of large, established companies succeeding with digital decisioning to illustrate the key principles of the approach. Whether you are using machine learning to drive operational efficiency, improve customer satisfaction, manage risk, or reduce fraud, digital decisioning will help you succeed.
9:55 am - 10:15 am
In a climate where 85% of data science projects fail, moving beyond the hype into the plateau of productivity necessitates understanding what differentiates successful from unsuccessful analytics initiatives. This talk takes an inside-out view of how analytics-driven organizations operate differently and provides insight into how you as a leader can harness their best practices.
As predictive modelers, we love advanced machine learning techniques such as neural networks, deep learning networks, and decision tree ensembles because the accuracy they can achieve can be significantly better than linear methods, such as linear and logistic regression. However, these methods do not offer the advantages of linear methods for model interpretation and diagnostics. Which variables are the most important in the model? How stable are the model parameters? How stable are the model predictions?
This talk will describe the use of random permutation to uncover and describe model input and model prediction sensitivities. Techniques such as Breiman’s “permutation importance” and the use of bootstrap sampling to uncover sensitivities will be discussed and will be applied to models built from data drawn from customer analytics.
9:30 am - 9:50 am
In this talk, we will explore production level machine learning application at Facebook scale and the lessons learnt in developing and deploying ML applications across Ranking & Recommendation, Computer Vision, and NLP domains.
We will learn the challenges across Data, Feature processing, Model training and serving at Facebook scale. The privacy and security implication and the continious integration / continious deployment consideration for ML applications.
9:55 am - 10:15 am
Machine Learning is emerging as a key enabler in building next generation products. Machine learning accelerates the product lifecycle. In this session, Walmart eCommerce's Director of Product Management Kunal Desai will explore two case studies, one where machine learning helped identify customer problems and the other where it helped scale a product from 1 to 100.
10:25 am - 10:45 am
Lack of trust in artificial intelligence / machine learning is pervasive throughout society. And the greatest areas of distrust are associated with AI’s potential impact on jobs. This lack of trust contributes to the under-utilization of collaborative AI systems and severely reduces their possible benefits. In this talk, Clayton discusses how to develop and launch AI systems to ensure that knowledge workers trust and ultimately use the system’s insights and recommendations.
10:50 am - 11:10 am
Success stories abound that extoll the use of machine learning and analytics in a wide variety of fields and on a wide swath of problems. But there are valuable lessons to be learned from what didn't work, whether a spectacular failure or just bumps in the road. In this talk, we describe real-world examples, some anonymous and most from the speaker's decades of analytical consulting. We will describe pitfalls, misconceptions, and uncertainties commonly encountered by companies in their path towards adoption of analytics that led to failure, lost time, and wasted investment.
Optimizing product features, promotion campaigns, and machine learning model hyperparameters is advantageous because effective configurations can improve outcomes, such as engagement, performance, and quality. However, it is often challenging to identify superior configurations because the space of possible configurations is vast, and the resources available for searching the space is limited. This talk introduces Ax, an open source platform for optimizing experiments using multi-armed bandit and Bayesian optimization, including how it has been successfully applied to a variety of product, infrastructure, and ML applications. By automating the process of identifying effective configurations via adaptive experiments, Ax enables product managers, developers, and analysts to efficiently get the most out of their software.
Also sign up for Clinton Brownley's workshop: Machine Learning with Python: A Hands-On Introduction
10:25 am - 10:45 am
All retailers want to know their target buyers better. However, understanding the past and present of their interactions simply isn’t enough these days and predictive analytics is the next step to better understanding their customers.
In this session, topics of discussion will include, yet will not be limited to:
- How ML can enable price optimization, product placement and assortment selection
- Using machine learning algorithms effectively for generating suggestions for substitute and complimentary items
- Using deep learning and reinforcement learning to improve order forecasting
- Utilizing optimization algorithms to reduce store costs by optimizing replenishment cycle and safety stock
- Scaling algorithms to generate recommendations for individual stores and to monitor their performance
10:50 am - 11:10 am
We developed a brand new reinforcement learning based approach to address one of the biggest challenges in email marketing - maximize engagement (CTR) and thus conversions. We have developed an agent that intelligently learns from previous campaigns and prescribes target population that better respond to a future campaigns. We refer to this agent as "PE" (Prescriptive Engine). Underneath the hood PE solves Multi-Arm Bandit problem/Exploration-Exploitation dilemma. In this session we will share details about the underpinnings of PE and how PE has been put into action at Samsung.
The three most important analytic innovations I’ve seen in (35 years of) extracting useful information from data are: Ensemble models, Target Shuffling, and Awareness of Cognitive Biases. Ensembles are competing models that combine to (very often) be more accurate than the best of their components. They seem to defy the Occam’s Razor tradeoff between complexity and accuracy, yet have led to a new understanding of simplicity. Target Shuffling is a resampling method that corrects for “p-hacking” or the “vast search effect” where spurious correlations are uncovered by modern methods’ ability to try millions of hypotheses. Target shuffling reveals the true significance of a model, accurately assessing its out-of-sample precision. Lastly, the increased understanding of our Cognitive Biases, and how deeply flawed our reasoning can be, reveals how projects can be doomed unless we seek out — and heed — constructive critique from outside.
Ari Kaplan will talk about his real-life Moneyball experiences of disruption in Major League Baseball front offices - and how artificial intelligence will disrupt every business industry. Having helped lead the adoption of data science throughout baseball, including creating the Chicago Cubs analytics department, he will lead lively discussion on how winning in baseball translates to winning across other industries, overcoming cultural resistance, and doing analytics at scale and velocity to win the race.

12:45 pm - 1:05 pm
In this session, Rich will discuss how Google approaches using AI and machine learning within the enterprise. He'll share how Google staffs and structures AI and machine learning teams, specific processes and governance of enterprise projects, and examples of AI and machine learning use-cases.
In addition to a high-level view, he'll deep dive into a number of these use-cases to understand them and their wider applicabilities.
1:10 pm -1:30 pm
In this presentation, we will share with the audience our work at AI at LinkedIn and cover what it takes to launch AI products at scale by way of a few examples, such as building a machine learning platform at scale for ML engineers at LinkedIn, and more.
Marketing isn't just any more about predicting who's more likely to buy a product, it's about identifying which customers are more likely to be persuaded by advertising. Traditional response models often target shoppers that would have bought a product anyways. Ib contrast, uplift modeling focuses on maximizing incremental sales by only targeting those customers that have a high likelihood of making a purchase if they receive an offer.
This session will cover how Sam's Club leverages this highly effective machine learning technique, and what it takes to develop a culture of experimentation and build out a contact and response history from numerous tests, which serves as a foundation for the development of uplift models.
12:45 pm - 1:05 pm
Fandango is the ultimate digital network for all things movies serving more than 60 million unique visitors per month across the entire movie life cycle. In this talk we will discuss Fandango360, Fandango's B2B platform that allows studio marketers and partners reach fans of their upcoming movies with targeted interactions across popular social media and advertising platforms. We will highlight some of the technical, machine learning algorithmic and application-centric novelties behind this endeavour and will conclude the talk with some of the performance marketing results, insights and learning stemming from hundreds of theatrical marketing campaigns run on the platform.
1:10 pm - 1:30 pm
In the programmatic AdTech space, the trend of header bidding explosion has put the strain on both Supply Side Platforms (SSPs) and Demand Side Platforms (DSPs). In many cases, 95% of bid requests from SSPs do not get a bid response from DSPs and DSPs hate processing so much junk. The result is ever-increasing infrastructure (AWS) costs for both sides of the business.
In this session, attendees will learn how machine learning techniques can be applied to millions of rows of data to predict which bid requests are unlikely to receive a bid response, and how even a minor improvement of 0.4% in average bid response rates can result in incremental revenue of $100,000 or more.
1:40 pm - 2:00 pm
Data Science provides a company the opportunity to create tremendous competitive advantages. Often times, Data Science applications within a company's product suite can differentiate it from the industry at large. This presentation covers some of the key strategies and concerns that a company must consider when pursuing the development of intellectual property for competitive advantage. Here we look at key considerations such as: what makes Data Science innovations patentable, when to consider patenting versus trade secrets, and how to identify areas where innovation will provide the most advantages for a company.
2:05 pm - 2:25 pm
Many analytic models never get the data they need to be successful; many analytic models that do are never deployed successfully into operations; and, many deployed models never bring the value they promised to stakeholders. In this talk, we give a framework for those leading analytic or AI projects or interested in leading these types of projects in the future that improves the odds for overcoming these and related challenges. We also discuss the results of a survey about some of the common reasons analytic and AI projects fail that underpins the framework.
Usually, a campaign's incremental sales or profit margins are used to evaluate the performance of marketing programs. The incremental sale or margin is : S/M(target group) - S/M(control group). Here, we have developed a predictive model for
max[S/M(target group) - S/M(control group)]
by using personalization data and machine learning methods. The first step is to develop the predictive baseline model at individual level:
P[S/M(control group)] = P[probability]* P[amount/purchaser]
which is for the estimation of the control group. Similar models are built for the target group. The selection of the target population in the future campaign is based on the maximum of the difference.
1:40pm - 2:00 pm
The cybersecurity market is now forecast to reach USD 289.8B by 2026 but the proper application of machine learning, deep learning, and AI to handle 500,000 new unique threats per day is imperative to ensure you're not a target. An appropriate development process examining the risks including Deep Fake (images, videos, and text), Model Hacking (Adversarial Machine Learning attacks) and Explainability of complex algorithms are key to building trust in the final security application. Learn how to protect yourself against these risks as you develop your algorithms, and what steps you can take to minimize your vulnerability.
2:05 pm - 2:25 pm
To create a foundation of expertise in data science, the National Energy Board of Canada (NEB), now the ‘Canada Energy Regulator,’ held cross–organizational data science workshops aimed at helping teams build capacity in data analytics, experimentation culture and evidence-based decision making. In this talk, industry expert Shingai Manjengwa of Fireside Analytics will cover the approach her firm implemented for the NEB, for both “hands–on” data science training, including computer programming, as well as an on–line version of the training that now has over 500 learners registered and a completion rate of 80%. This case study showcases a novel professional development and training approach to building these capacities. Find out how they did it and how you increase capacity in your organization.
2:35 pm - 2:55 pm
Machine learning and product management are two of the hottest things in tech right now, but putting them together requires reflection on the specific skills, techniques, attitudes and responsibilities for PMs when it comes to ML-driven products. Machine learning is not 'technology as usual' and so product managers need to adapt by a) understanding how the technology works, b) learning how to communicate an ML-centric value proposition to diverse stakeholders, c) adjusting how the MVP is defined and d) not becoming so enamored with the ML that the non-ML stuff is forgotten.
3:00 pm - 3:20 pm
You may have heard the quote, "culture eats strategy for breakfast"; even the best strategies can fall flat without the right energy in execution.
In this session, you'll hear about how AppFolio's Analytics & Research Community has inspired expanded leadership engagement, deeper cross-functional collaboration, and new ways of thinking across the business. Join us for this session if you'd like to learn more about how to build and nurture a fun and impactful Analytics culture, and avoid the sentiment of "just another meeting".
Randomized experiments allow us to determine the overall treatment effect of a program (e.g. marketing, medical, social, education, political, economic). Uplift modeling takes a further step to identify individuals who are truly positively influenced by a treatment or intervention through machine learning and predictive modeling by uncovering heterogeneous treatment effects in available data. This technique enables us to identify the “persuadables” and thus optimize target selection in order to maximize treatment impact. This important subfield of data science or business analytics has gained tremendous attention in recent years in application areas such as personalized marketing, personalized medicine, political election, and healthcare programs with plenty of publications and presentations from both industry practitioners and academics across the world.
However, business and medical applications often involve more than one treatment. Additionally, there are often budget and quantity constraints involved. This talk will review current uplift modeling methodologies, extend predictive modeling to multiple treatment situations, bridge the gap between predictive analytics and prescriptive analytics by introducing the mathematical problem for treatment optimization, and propose various solutions to both deterministic and stochastic optimization problems. Examples from the retail industry will be used as an illustration. While the talk is geared towards marketing type applications (“personalized marketing”), the same methodologies can be readily applied in other fields such as insurance, medicine, education, political, and social programs.
Companies are frequently faced with large amounts of unstructured text data, like forum comments or product reviews. Important trends can emerge in these datasets, but it can be time-consuming to read through comments, and keyword matching frequently misses critical nuances. We'll discuss how we've approached this problem at Google using Natural Language Processing, with examples of the approach applied to open datasets. We'll explore how this fits into the ML project lifecycle, with examples of common pitfalls. Finally, we'll highlight how to use this technology as part of a "human in the loop" approach to supercharge your existing team members.
Predictive Analytics World for Business - Las Vegas - Day 2 - Wednesday, June 3rd, 2020
Drawing from his experience as the chief data and analytics officer at three different companies, A. Charles Thomas – now chief data and analytics officer at General Motors – will share insights and lessons learned from both sides of the unique, two-pronged role he plays at GM.
First, Charles' team leverages analytics to enhance GM's traditional businesses, such as selling vehicles, OnStar, Warranty, SiriusXM, and others. The team generates insights to drive billion-dollar improvements in functions such as manufacturing, HR, Marketing, and Digital.
Second, Charles' team also drives revenue from their unique access to tremendous quantities of vehicle data. This includes direct licensing of connected vehicle data (e.g. GPS data to traffic and parking apps, media, retail, and insurance companies), as well as using these data to create new businesses in insurance, fleet management, and others.
In this keynote address to both the PAW Business and PAW Industry 4.0 audiences, Charles will share his unique insider's vantage.
Rexer Analytics has been surveying analytic professionals for over a decade. In 2020, over a thousand people from around the world participated in the 9th Data Scientist Survey. In this PAW session, Karl Rexer will present highlights of recent survey results and discuss trends from the past decade.
Highlights will include:
- Trends in algorithm usage, software adoption, and job satisfaction
- Key challenges reported by today’s analytic professionals
This presentation is about making machine learning models and their decisions interpretable, and why that’s important and valuable
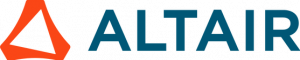
Track Co-Chair: James Taylor
The enormous effort devoted toward applying machine learning to mimic human decision-making may be misplaced and even counterproductive for many organizations. For knowledge work, in particular, we should not strive for systems to replace humans, since we run the risk of reifying the cognitive and organizational biases that already exist. Instead, we should harness the power of computers to perform tasks that human experts are not very good at accomplishing (such as situational awareness), leaving more time in the workday for human experts to do what they are good at (thinking creatively and identifying causal relationships). And by building joint human-machine platforms, we can efficiently share knowledge across an organization and prioritize what additional information to collect. This approach can also ease the transition from today’s workplace to a future one, by starting with expertise-driven causal explanations and by requiring advanced analytic methods to be understandable.
In addition to describing a future workflow in which we leverage the best of human and machine intelligence, this presentation also points out the challenges in moving to this future. Specifically, the presenter will discuss key technical and cultural gaps that will need to be overcome if we are to get greater value out of the data we possess and collect and to enable data-driven decision-making at scale. These gaps represent priority areas for research funding, which business leaders should support.
Explanations enable human interpretation of complex models and are increasingly demanded to promote actionability and abide by regulatory and ethical guidelines. But what can you really learn from the vast suite of explainers available today, and which tool is best for your customer’s purpose? We discuss some common algorithms, the problems they were designed to address, and their suitability for "off label" uses frequently requested by business stakeholders.
9:25 am - 9:45 am
Predictive analytics has been contributing to address some of the world’s most challenging social problems. Predictions have been successfully driving decisions in many social domains such as crisis response and disease outbreak, economic empowerment and financial inclusion, health and hunger, student education and teacher productivity, security and justice, climate change and adaptation, and many others.
In this talk, Prof. Bari of New York University and his team will highlight the societal benefits of predictive analytics’ algorithms that can create both business opportunities and social impacts to help both developed and developing economies. The talk will answer questions such as: Can predictive analytics fight loneliness? can algorithms detect malnutrition? how do recommendation systems help refugees integrate? what are the capabilities of predictive analytics that can address climate change? how can image recognition detect skin cancer through smart phone images? how can deep learning be applied detect road outages from satellite images after natural disasters? how can predictive analytics be leveraged to maximize student achievement? How can natural language processing be used to detect student distress before the school notices?...
The talk will also address the ethics, risks, and limiting factors, and how to mitigate them to realize a social impact. Prof. Bari was formerly with the World Bank Group and has been holding the role of AI advisor for a leading international organization. Julian Smith is a master’s candidate and researchers in computer science at the courant institute of mathematical science of New York University. His research focuses on using predictive analytics to address climate change.
9:50 am - 10:10 am
Data science and predictive analytics play a key role in churn management by empowering companies to identify at-risk subscribers, as well as determining the incentive or intervention with the highest likelihood of leading to customer retention.
In this talk, I will present the use case of Philips Lifeline in managing subscribers of our medical alert service. We identified at-risk cohorts by predicting their likelihood of churn and applied A-B testing of a cost-efficient intervention. Using predictive analytics to leverage the efficient use of our intervention, we reduced the churn rate by 50% in subscribers with a high predicted risk of churning.
10:20 am - 10:40 am
AI is the new electricity. It is fundamentally and radically changing the fabric of our world. But like any new technology, it has a dark side: machine bias. If unchecked, machine bias leads to biased products and services which may discriminate against some of your customers, citizens, or employees based on their race, gender, age, etc. Bias is also bad for organizations: it can lead to missed opportunities, lost consumer confidence, reputational risk, etc. Machine bias, therefore, is organizational risk. This session will help you learn about machine biases: how they emerge, why they are dangerous, and how mitigate them.
While decision trees play a central role in contemporary data science applications, they are almost always used in large ensembles combining hundreds or even thousands of trees. The gradient boosting machine (which leverages hundreds to thousands of small trees) is perhaps the all-time most popular learning machine and made its recent reputation displaying superior performance across a number of Kaggle competitions; Random Forests (which leverages hundreds of large trees) is well known for its feature selection prowess especially in the context of huge numbers of potential predictors. Unfortunately, the excitement generated by the power of these modern learning machines has induced the field of data science to neglect the value and dramatic effectiveness of their direct predecessor, the single decision tree. In this presentation, we step back in time to review what made the single decision tree such a revolutionary analytical tool and we present a number of applications in which the single decision tree is more effective and more appropriate for the problem at hand than subsequent multi-tree methods. Examples are drawn from e-commerce consumer behavior, consumer insurance billing, detecting undesirable differences between two or populations, and the interpretation of complex models.
10:20 am - 10:40 am
Over 160 Billion digital breadcrumbs teach us about people's behavior online and offline every day. Harnessing these behavioral signals, we can model and predict behavior and changing states of need and consumption. In this case study, we predict consumers whose subscriptions are up for renewal and are highly likely to churn (cancel their subscription). Can we correctly identify them based on past digital behavioral patterns? Can we learn anything about them in order to understand their motivation for this behavioral change, and possibly change their minds? This fascinating journey through online behavioral data and its uses will answer these questions.
10:45 am - 11:05 am
Wix is a freemium website builder with over 4 million active paid subscriptions. To retain customers, we built a model which predicts how likely each user is to churn. The model is live, and helping us save users. In this talk, Wix's Gil Reich will share this journey and their top lessons, including the how and why of establishing an end-to-end model, treatment, and feedback.
How many .edu addresses are in your inbox right now? As organizations pursue digital transformation strategies, challenges related to finding and retaining analytical talent, objectively assessing the relevance of new, and emerging technology and engaging in deep and meaningful innovation with eventual payback are common to all sectors of the economy. Deep, collaborative partnerships with universities can help mitigate many of these challenges. This is all the more true because data science itself has given rise to a new "entrepreneurial university" paradigm. Dr. Priestley is an academic Associate Dean, who worked for organizations like Accenture and VISA EU, and now manages corporate partnerships with the likes of Blue Cross Blue Shield, Emerson, Equifax, and GE, as well as fire departments and law enforcement. She will discuss the ways that organizations should be thinking about working with universities, but typically don't — including research, innovation, "externships," training options, recruitment, and other strategic relationships. After this session, you will never look at universities the same way again.
Metis Corporate Training rests on Kaplan’s heritage of 80 years of instructional excellence. We began by operating the only accredited data science bootcamp - awarded by the Accrediting Council for Continuing Education & Training (ACCET) - and producing graduates who consistently impressed their hiring managers. Those hiring managers asked us to provide their teams with the same quality training as our alumni, so we began our Corporate Training division. Fast forward, we are now the trusted data science and analytics training provider for Fortune 500 companies, as well as cutting edge startups, around the world. Learn about our story and how to get your teams the technical training they will truly appreciate.
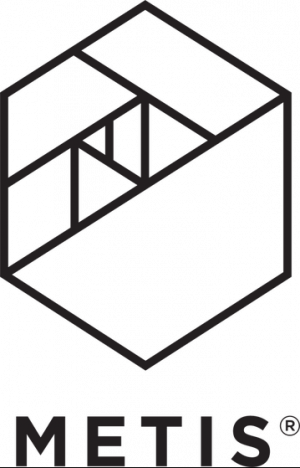
No one wants to go to jail. Making decisions based on machine learning models offers new and exciting ways to be convicted -- in court and in the court of public opinion. Models that encode bias and automate discrimination are illegal or at least unethical. Demonstrating the compliance of a model is a hurdle that regulated industries must cross for every model. Even models that seem fine to you and your regulator might sound awful when described in the media. Ethics and compliance are as important to your success as data quality, MLOps, and operationalization. This panel will discuss ethics, bias, regulatory compliance, explainability, and much more to help keep you and your models out of jail.
1:25 pm - 1:45 pm
As organizations transform into data and analytics centric enterprises, the talent pool is barraged with demands for talent to build innovative, agile data solutions. Strategic, intentional partnerships with universities can help you develop and acquire graduates with the skills to implement not only state-of-the-art data science but also compelling narratives from data, collaboration, and creativity. Join us to learn how HanesBrands' partnership with Elon University has yielded a pipeline of premier talent through research, national Champion® analytical challenges, on-campus internships, and customized sales online learning modules.
Solving a data science problem is an iterative exercise. It requires running experiment after experiment — trying new approaches with different parameters and lots of data. To manage this complexity, it is very helpful to have a platform to build reusable workflows that can be tracked.
Kubeflow Pipelines is a component of the Kubeflow open-source project, focused on building and deploying portable ML workflows on Docker containers. In this session, the audience will learn about KubeFlow Pipelines and how it can help improve reuse and reproducibility of the machine learning process.
1:25 pm - 1:45 pm
Although many investors may view mergers and acquisitions as attractive to an organization, for many employees, these transactions are viewed as threatening, unstable, and downright scary. In addition to lack of trust in leadership and a proper culture fit; reduction in force, down leveling, loss of certain company benefits are some of the typical fears of employees involved in M&A. As such, many employees take control of their “destiny,” and simply exit. This presentation is focused on how an organization facing acquisition can identify the drivers of employee attrition and implement proactive measures to prevent employees from leaving.
1:50 pm - 2:10 pm
Employer investments in employee health and wellness represent an increasingly expensive component of total rewards. The efficacy of these investments is commonly assessed in terms of their impact on measures of employee health, their direct impact on total labor costs and on the labor market competitiveness of the offer. Unfortunately, these outcomes do not reflect the full economic value of health and wellness investments.
This session will demonstrate how applying advanced workforce analytics can help measure the actual workforce impact of these investments and their true economic value. The presenter will highlight examples drawn from actual client work where predictive modeling methods applied to integrated sets of health and HRIS workforce data have helped leaders to quantify the workforce impact of health and wellness programs and estimate their ROI. The modeling can also reveal how work environment affects the trajectory of health claims and risks. Finally, the session will speak to the modeling methods used and the importance of using a coherent economic lens to inform modeling strategy and interpretation of results.
Data analytics and the associated data management best practices enable conversion of raw disparate data to enrich valuable information as part of the digital transformation initiatives. The vision and mission statement together with well-formed DataOps practices aid the formulation of the key business objectives and guide the tactical and strategic intelligence objectives, including applicable and performant predictive analytics. This talk will focus on how the DataOps mechanisms such as data catalogs, data lineage and, data fusion leading to near-transparent self-service analytics and MLOps effectively enable improved decision-making and thereby, the operational efficiency (and/or data monetization) of the organization.
2:20 pm - 2:40 pm
Segmentation and Profiling is a technique to understand how segments impact various business metrics. Typically, analysts do it using pivot tables and drill-downs to understand significant trends and trend changes. This is a laborious activity, especially if it needs to be extensive. But, by inventively using Association Rules Mining technique, we can perform automatic segmentation and profiling and extract trends and trend changes over large data sets. Changes in trend across time intervals can be automatically detected and reported in an extensive manner while saving time and effort.
2:45 pm - 3:05 pm
Making machine learning a first-class citizen in application programming is one of the "holy grails" of the domain. Packaging ML into abstractions practical for everyday development involves challenges at different layers of the programming and research stack, as well as developing new layers and new kinds of abstractions. We present a vision of how such a stack could look like, and recommend directions of necessary work to make it a reality.
2:20 pm - 2:40 pm
Under EU261 directive, airplane passengers have the right to be compensated if their flight is sufficiently delayed. GIVT helps passengers file such claims. Every claim needs to be verified as there are various conditions that can invalidate it: extreme weather, strikes, bird hit, etc. In this talk, I will describe a machine learning system which replaces manual verification of claims.
2:45 pm - 3:05 pm
"How much will I sell?" is a simple question with a complex answer. Stocking the right amount of a product in a warehouse is like navigating between Scylla and Charybdis: keep too much on hand and pay warehousing fees and the opportunity cost of holding; keep too little on hand and lose out on potential sales.
In this talk, we will present an overview of ChannelAdvisor's demand forecasting system for e-commerce brands and retailers, which helps customers understand sales forecasts, allowing them to manage their inventory more effectively.
Post-Conference Workshops - Thursday, June 4th, 2020
Full-day: 7:15am – 2:30pm
This one-day session reveals the subtle mistakes analytics practitioners often make when facing a new challenge (the “deadly dozen”), and clearly explains the advanced methods seasoned experts use to avoid those pitfalls and build accurate and reliable models. Click workshop title above for the fully detailed description.
Full-day: 8:00am –3:00pm
Gain the power to extract signals from big data on your own, without relying on data engineers and Hadoop specialists. Click workshop title above for the fully detailed description.
Full-day: 8:00am –3:00pm
This workshop dives into the key ensemble approaches, including Bagging, Random Forests, and Stochastic Gradient Boosting. Click workshop title above for the fully detailed description.
Full-day: 8:00am –3:00pm
During this workshop, you will gain hands-on experience deploying deep learning on Google’s TPUs (Tensor Processing Units) at this one-day workshop, scheduled the day immediately after the Deep Learning World and Predictive Analytics World two-day conferences. Click workshop title above for the fully detailed description.