Predictive Analytics’ Killer App: Retaining New Customers
By Eric Siegel, Ph.D.
Conference Chair, Predictive Analytics World
There is a goldmine of growth available to certain lucky businesses: those with many one-time customers. To these companies, with a large portion of new customers never returning, the flow of passers-by presents a unique opportunity.
How can this potential be tapped? What can be done to entice one-time customers to stick around? The standard approach is to pursue improvements in business operations, such as better products, greater relevancy in marketing or more personalized service. Usually, a significant improvement in growth requires a significant investment in one or more of these areas. But, given the steady flow of one-time customers, is there a way to get more for less?
Yes, there is a clever way to capture many of these prospects for a relatively small increase in expenses. If you predict which first-time customers won’t return, you can target them with an aggressive conversion campaign, enticing those that would otherwise leave with a heavy discount or free trial. In this way, you turn the first date into a long-term relationship.
The challenge is in the predictive targeting. Without knowing ahead of time which new customers will turn out to be hit-and-run customers, a costly retention campaign sacrifices too much revenue from the customers who were going to return anyway. In other words, if you offer every new customer a rebate on her or his second purchase, those destined to come back will simply pay that much less when they do.
Predictive modeling does the trick. A predictive model tells you which new customers are likely to return and which are probably one-timers. The model is created with data mining methods that “learn” from the collective experience of your company contained in your sales records. The model then applies what has been learned to produce a predictive score for each new customer in real time.
In this way, new customers you would otherwise never see again are targeted and enticed to stay. Because you don’t waste the retention offer on new customers likely to return, the numbers work out very well. The growth rate and medium-term profits potentially skyrocket, and immediate-term profits are not put at risk.
Targeted Rebates Increase Growth without Sacrificing Revenue
Figure 1 illustrates this process. Consider an online retail store that attracts many one-off customers with Internet advertising. New customers who have just made their first purchase enter from the left. These customers are then routed in one of two directions, according to a predictive model. Customers likely to return without any additional incentive are left alone, while customers unlikely to return are targeted with an aggressive conversion campaign, such as an email coupon offering 30 to 50 percent off their next purchase.1 If 80 percent of a company’s new customers are presently one-timers, capturing just three percent of this massive flow results in a 12 percent increase in the overall customer growth rate.
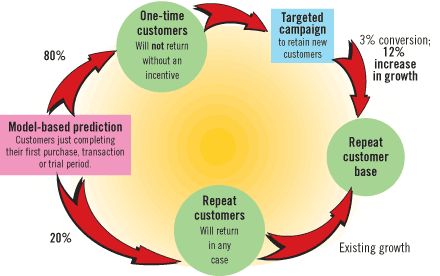
The retention offer must be delivered immediately after a new customer period. If you wait to see whether the customer defects, it will already be too late. The exact timing of the retention campaign depends on the business model, as detailed in Figure 2. For some businesses, this moment of opportunity comes at the transition between clearly defined stages of the customer lifecycle. For example, retail customers are at a “stay or go” crossroads immediately after the first purchase, so offer them the coupon right away. In contrast, subscription-based services often start with a brief trial period. Will the customer cancel or maintain the subscription after the trial ends? Other types of businesses, such as free services, may require creativity in delineating the precise moment when a new at-risk customer should be targeted for retention.
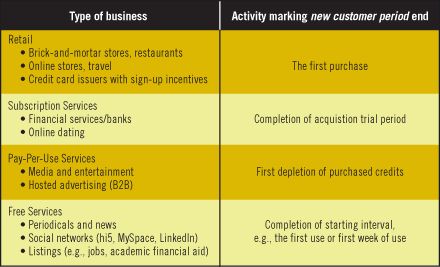
Retention Campaigns to Increase Profits
Predictive analytics is the “magic ingredient” needed to make the numbers game work. You can’t afford to deploy an aggressive rebate campaign without knowing which customers will return anyway. But, without literally relying on magic, how is prediction possible?
Predictive modeling leverages your sales and customer records to discover patterns that distinguish one-time customers from repeat customers. Predictive analytics’ core methodology automatically determines which attributes of a new customer, in combination with everything you observe about the first purchase or transaction, make the difference. This often comes in the form of business rules, such as the following fictional example:
If the new customer comes to the Web site off organic search results,
and buys more than $150 on the first transaction,
and is male,
and has an email address that ends with .net,
then this customer is three times as likely to be a returning customer.
I explore predictive modeling in greater depth in the article, “Predictive Analytics with Data Mining: How it Works.” http://www.predictionimpact.com/predictive.analytics.html. Go and check it out for a bite-sized dose of the inner mechanics of predictive modeling.
Benefits of Retaining New Customers
Predictively targeting a retention campaign toward customers at risk for defection is not a new idea, but given a high rate of one-time customers, there are advantages to targeting new customers rather than tenured ones.
- More opportunities. A high bandwidth of new one-time customers presents a great wellspring of prospective repeat customers. This wealth of ROI opportunity dwarfs the frequency of defecting tenured customers.
- More rows of data. This high bandwidth also provides plenty of corporate experience in the form of data, one row per customer, recording who stayed and who left, which amounts to many more examples with which to develop predictive models.
And, you get the best of both worlds because retaining new customers also gains the benefits of predictive retention campaigns in general, which include:
- Higher ROI. As marketing lore consistently states, retention is less expensive than acquisition. That is, you can increase growth with less cost by retaining existing customers rather than acquiring new ones.
- More loyal customers. Likewise, increasing the number of longer-term repeat customers with retention, rather than new customers with acquisition, provides a more valuable base of loyal customers. Loyal customers are known to have many advantages, including higher profit margins, more frequent purchases and lower sensitivity to pricing.
- More columns of data. Much more information is available about each existing customer targeted for retention than each prospective customer targeted for acquisition. Since business has already been conducted with existing customers, you know their profile, billing information and, to some degree, their buying behavior and/or product-use history. These tidbits, each filling out a column in the database of customers, are vital for developing an effective predictive model.
The Payoff
Just slightly tapping into the high flow of one-time customers increases the growth rate significantly. For example, as depicted in Figure 3, if 80 percent of new customers are one-timers, retaining just three percent increases growth by 12 percent.
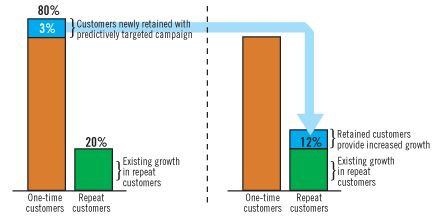
The revenue numbers further support the value of predictively retaining new customers. Profits often suffer by directing a retention campaign to all new customers. For example, say you send a hefty coupon for 50 percent off the customer’s next purchase to all new customers. Even if you assume this campaign has a high response rate of 20 percent, due to great value to the consumer, the overall effect is to decrease rather than increase revenue, because customers who were going to return anyway will exploit the coupon and simply pay that much less.
However, if the same campaign is targeted only to those customers predicted to not return, the overall profit increases. Assume your business currently retains 20 percent of new customers. Predictive modeling may discover a series of subsegments (such as that in the fictional business rule example) that together identify 60 percent of new customers, each of whom are 40 percent more likely than average to return. This is a realistic result, and a valuable one at that, enough to overcome the loss in revenue that comes from offering a rebate by achieving a significant forecasted increase in retention, as shown in Figure 4.
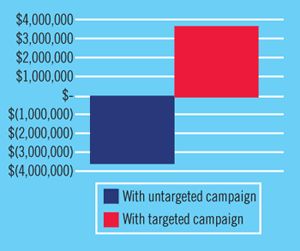
Like all models, the forecast model to calculate the revenue results (not to be confused with a predictive model, which provides individual predictive scores for each customer) works under a set of simplifying assumptions. For example, we assume customers destined to return will make the same purchases and simply pay less if given a rebate coupon. Furthermore, the retention campaign’s hit rate, presently assumed to be 20 percent, should be explicitly tested before relying on forecasted results. Although your campaign can be quite aggressive, given the ability to target only those customers most at risk, the success of the campaign depends on other factors, such as the nature of the product – is it a product people tend to buy repeatedly or only a few times in one’s life?
To examine the set of assumptions and full forecast arithmetic and to try plugging in some of your own numbers, see the spreadsheet at www.predictionimpact.com/retention.
Having read this article, you are a new customer. If you haven’t clicked for more information on the URL above, I predict you are on the way out the door. Wait one moment! In order to retain you as a repeat consumer of predictive analytics, I’ll drive home the benefits and take-aways. If you are seeing an abundance of one-time customers, you are faced with a tremendous growth opportunity, and you already have an invaluable strategic asset at your fingertips with which to tap this potential – the data recording these customers’ profiles and buying behavior. A predictive model developed over this data targets retention campaigns and increases profitability.
Reference:
- This method is, in effect, a form of price sensitivity estimation and dynamic price selection, since the cost of a customer’s second order is optimized for each individual customer.
About the Author
Eric Siegel, Ph.D., is a leading consultant and former Columbia University professor who makes machine learning understandable and captivating. He is the founder of the Predictive Analytics World and Deep Learning World conference series, which have served more than 17,000 attendees since 2009, the instructor of the acclaimed online course “Machine Learning Leadership and Practice – End-to-End Mastery”, a popular speaker who’s been commissioned for more than 110 keynote addresses, and executive editor of The Machine Learning Times. He authored the bestselling Predictive Analytics: The Power to Predict Who Will Click, Buy, Lie, or Die, which has been used in courses at more than 35 universities, and he won teaching awards when he was a professor at Columbia University, where he sang educational songs to his students. Eric also publishes op-eds on analytics and social justice. Follow him at @predictanalytic.