Conference Day 1: Monday, April 4, 2016
8:00-8:45am • Room: North Registration
Registration
8:00-8:45am • Room: Salon 8 & 9
Networking Breakfast
8:45-8:50am • Room: Golden Gate A
Conference Chair Welcome
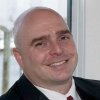
COO
Rising Media, Inc.
8:50-9:40am • Room: Golden Gate A
KEYNOTE:
Weird Science: How to Know Your Predictive Discovery Is Not BS
"An orange used car is least likely to be a lemon." At least that's what was claimed by The Seattle Times, The Huffington Post, The New York Times, NPR, and The Wall Street Journal. However, this discovery has since been debunked as inconclusive. As data gets bigger, so does a common pitfall in the application of standard stats: Testing many predictors means taking many small risks of being fooled by randomness, adding up to one big risk. John Elder calls this issue vast search. In this keynote, PAW founder Eric Siegel will cover this issue and provide guidance on tapping data's potential without drawing false conclusions.
[ Top of this page ] [ Agenda overview ]
9:40-10:00am • Room: Golden Gate A
Diamond Sponsor Presentation
Enabling Data Science for Lambda, Lakes and Bases
Data is managed in many different forms from relational and NoSQL databases, to large scale Hadoop data lakes or even high-speed real time data streams. Developing insights and machine learning solutions that have a measurable benefit for the organization requires "data scientists" to operate across lambda, lakes and bases. Oracle products allow data scientists to spend less time dealing with the challenges of managing data, simplifying their data preparation and modeling process. This means Oracle customers like StubHub can develop predictive analytic solutions more quickly and deploy them into production to realize their benefit.
10:00-10:30am • Room: Salon 8 & 9
Exhibits & Morning Coffee Break
10:30-11:15am • Room: Salon 3 & 4
Track 1: Uplift modeling
Case Study: U.S. Bank
Uplift Modeling: Optimize for Influence and Persuade by the Numbers
Data driven decisions are meant to maximize impact - right? Well, the only way to optimize influence is to predict it. The analytical method to do this is called uplift modeling (aka, persuasion modeling). This is a completely different animal from standard predictive models, which predict customer behavior. Instead, uplift models predict the influence on an individual's behavior gained by choosing one treatment over another.
In this session, PAW founder Eric Siegel provides an introduction to this growing area to prepare newcomers for this PAW event's other two sessions and full-day training workshop on the topic:
- Session with Patrick Surry
- Session with Jim Porzak and Ming Ng
- Full-day training workshop with Kim Larsen
10:30-11:15am • Room: Golden Gate A
Track 2: Modeling Methods (Algorithms)
The Five Tribes of Machine Learning, and What You Can Take from Each
There are five main schools of thought in machine learning, and each has its own master algorithm - a general-purpose learner that can in principle be applied to any domain. The symbolists have inverse deduction, the connectionists have back propagation, the evolutionaries have genetic programming, the Bayesians have probabilistic inference, and the analogizers have support vector machines. What we really need, however, is a single algorithm combining the key features of all of them. This talk describes my work toward this goal and speculates on the new applications such a universal learner will enable.
10:30-11:15am • Room: Salon 5 & 6
Track 3: Predictive Investing (VC)
Case Study: Microsoft Strategy
Predicting Startup Success: Finding the Unicorns among Wildebeests
In this presentation, Mukund from Microsoft Strategy will talk about predicting startup outcomes. Using data from over 74 public and private sources, we attempt to quantify our pipeline of startups, deal flow and portfolio of companies. The predictive analytics techniques we use are to help us determine which startups have a higher likelihood of outperforming the market. We use the research and analytics to help us source better companies, manage our pipeline of deals and help support their portfolio companies to scale and grow.
[ Top of this page ] [ Agenda overview ]
11:20am-11:40am • Room: Salon 3 & 4
Track 1: Analytics in Microsoft's
Move to SaaS
Case Study: Microsoft
Predicting User and Device Upgrade Issues Moving to Windows as a Service
The development of Windows 10 relied heavily on the newly created Windows Insider Program. In this Program several million Windows enthusiasts signed up to get early access to Windows 10 build. In exchange, these users agreed to have telemetry collected from their devices to aid in the development of Windows 10. In this talk I will illustrate the value of the Windows 10 Insider program with a case study: The telemetry collected from these Insiders covers a broad range of devices and configurations as well as usage patterns. Based on this data we could develop predictions that allowed us to predict which devices and users in the general population would have a successful upgrade experience and which devices and application configurations may run into upgrade problems. Among others, this allowed Microsoft to prioritize the steps needed to be taken to remove friction from the upgrade experience.
11:20am-12:05pm • Room: Golden Gate A
Track 2: Uplift Modeling
Case Study: Telenor
Applying Next Generation Uplift Modeling to Optimize Customer Retention Programs
Organizations must constantly work to drive greater retention and revenue whilst spending less money and using fewer resources. In this session, hear how the world's 7th largest mobile operator has applied next generation "uplift" modeling (i.e. "net lift" modeling) to optimize retention programs and seen results 36% better than those possible using traditional analytic practices. Impressively, these results were reached while, at the same time, slashing the cost of retention programs by a staggering 40% – making this an ideal fit for today's recessionary marketing requirements.
Uplift models are different from traditional modeling in that the approach measures and predicts the true incremental impact of marketing activity. Whereas traditional models only aim to predict "behavior", uplift models actually predict the incremental "change in behavior." Telenor's novel approach and application was recently featured in Forrester Research's popular new report "Optimizing Customer Retention Programs", where the approach was shown to achieve an 11-fold increase in campaign ROI when compared with existing programs.
See also the full-day uplift modeling workshop on April 7
11:20am-12:05pm • Room: Salon 5 & 6
Track 3: Predictive Audit Planning
Case Study: General
Electric
Advanced Analytics and the Corporate Audit Function
This session will discuss advanced analytics, the corporate audit function, and using data science as the enabler. The first half of the session will describe the role of audit to the organization, types of audits, how analytics have been used in the past, what we are trying today, and where we want to be. The second half will discuss a practical example, with cleansed data, to describe the use of competing models like GAM and Random Forests to predict different cycles for audit planning using mixed data types augmented with NLP to mine the narrative fields.
11:45am-12:05pm • Room: Salon 3 & 4
Track 1: Predictive Analytics for SEO and Online Marketing
Case Study: CanIRank.com
Predicting Online Marketing Success: Five Lessons Learned
Can we predict which website content will rank highly in search engines, go viral on social media, or earn links from bloggers and journalists? (Chair's note: Their results indicate "yes.") And along the way, can we learn enough about what factors drive online marketing success to make useful recommendations for traffic-hungry marketers? These are the questions we set out to address in a two-year odyssey to develop what ultimately became CanIRank, online marketing software that delivers actions rather than data. We'll explore lessons learned, including the unique challenges of modeling messy web data; ensuring customers want what you're trying to build, and model deployment in real-time web apps.
[ Top of this page ] [ Agenda overview ]
12:05–1:30pm • Room: Salon 8 & 9
Lunch in the Exhibit Hall
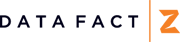
12:05–1:30pm • Room: Salon 5 & 6
Lunch & LearnUsing Apache Spark on the Mainframe to Reduce Fraud in the Financial Sector
A recent survey by IBM of banking and financial market executives found that only 56% believe their organizations are in reasonable control of fraud threats. Direct fraud charge-offs alone account for more than seven basis points of revenue for at least 70% of the institutions surveyed. Fraud and financial crimes remain a very expensive problem in the financial sector.
Real-time analysis – the ability to interdict a fraudulent transaction before it is settled – is key to reducing the cost of fraud, yet only 16% of the institutions in this survey have gone down this path.
For institutions that drive payment systems on the mainframe, new capabilities based on Apache Spark from IBM, DataFactz and Zementis open the door for real-time, in-transaction fraud detection and prevention in any industry that settles electronic payments. This lunch and learn will discuss how to make this happen.
12:30–1:00pm • Room: eZone, Booth 418
Book SigningRevised and Updated Predictive Analytics: The Power to Predict Who Will Click, Buy, Lie, or Die
Books are free to PAW Business attendees
1:30-2:15pm • Room: Golden Gate A
KEYOTE
Buy or Wait? Consumer-friendly Airfare Prediction or How the Bunny Saves You Money
Buying a plane ticket is a time-consuming and frustrating process that often leaves the consumer unhappy. Flight prices are less transparent and fluctuate more than almost anything else a consumer buys, even though airfare is one of the most expensive purchases for a typical family.
Our goal at Hopper is to bring more transparency to pricing, by giving consumers advice about where and when to fly -- and when to buy -- to save money on their air travel. We believe this helps consumers buy more quickly, with less effort, and ultimately be happier with their purchase decision. One of our key features is our "when to buy" advice: we'll watch prices for your trip and notify you when the price is right.
Recommending when to buy is tough for two main reasons: first is the airfare marketplace and its idiosyncrasies present unique analytical challenges, and second is that the prediction must be highly consumer-friendly: both easy comprehensible and immediately actionable. If we're too conservative and tell you to buy too early, we risk missing out on a better deal later, but if we're too optimistic and wait too long, you could end up paying more as prices rise towards your departure date. Because prices change at the whim of the airlines in unpredictable ways, it's impossible to know for sure. But this session will outline how we've overcome these challenges to help consumers save 10% on average, and up to 40% in some cases.
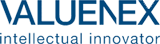
2:15-2:25pm • Room: Golden Gate A
Gold Sponsor Presentation
How Can We Find the Future on the RadarMap of Big Data?
Valuenex provides solutions for the future from massive amounts of documents and Big Data through unique methodologies, proprietary algorithms, and customized consultation services, with over a decade of experience in the industry. From this, the Valuenex Radar was developed, which uses over 60 million dimensions, compared to the 256 max dimensions of typical analytics tools in this field. This extraordinarily high amount of dimensions allows you to predict future scenes with precision. This is where the Valuenex methodology differs from typical Big Data Analytics tools based on Hadoop and MapReduce. The Valuenex Radar suite also provides helpful and original indexes such as document density, gravity trends, and data rankings. When technological documents of patents are applied, you can find future opportunities as white spaces, which are clearly and automatically defined. The Valuenex Radar can be applied in the finance sector, R&D strategy, detection in healthcare, and legal perspective planning.
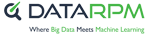
2:25-2:35pm • Room: Golden Gate A
Gold Sponsor Presentation
Cognitive Data Science for Predictions
As organizations compete with each other for driving amazing customer experiences, they are inundated with data explosion. With the much predicted shortage of data scientists and complexities of diverse tools, the call of the hour is cognitive data science. In this session, Ruban Phukan will outline how cognitive data science empowers companies to build data products for recommendations and predictions. The session will also cover use cases from Fortune 50, Fortune 100 based on cognitive data science for predictive maintenance and product recommendations.
2:40-3:00pm • Room: Salon 3 & 4

Case Study: Autodesk
Adopting Analytics - The Autodesk Journey
Autodesk's transition to a subscription based business model has caused the company to rethink how we interact with and engage our customers. The desire to have a granular understanding of our customers' needs and behaviors is paramount, and the chosen path to help achieve this goal is through advanced analytics. In a short period of time, Autodesk has identified and executed on numerous data science projects that have enhanced our operational capabilities to acquire, retain, and provide more value to our customers. This session will highlight what we've done, how we did it, and how we plan on doing more.
2:40-3:25pm • Room: Golden Gate A
Track 2: Understanding Viral Diffusion: Data Science at Mashable
Case Study: Mashable
The problem of predicting the popularity of content diffusing on social networks is a compelling, but difficult one--particularly when the structure of these networks is necessarily hidden. Through a variety of modeling techniques we'll discuss, at Mashable we've developed an accurate forecast for the future popularity of a wide range of content and use it to maximize editorial impact.
2:40-3:25pm • Room: Salon 5 & 6
Track 3: Omni-Channel
Case Study: CIBC
Driving the Omnichannel Experience with Predictive Analytics
In today's omnichannel world, customers utilize a combination of mobile, online, ATM, call center, branch, and email to meet their day-to-day financial and banking needs. Online and mobile banking continues to rise, and yet branches will remain. To strategically optimize the omnichannel experience, banks must adapt through Test, Learn, Fail Fast and Fixed Fast.
CIBC employed test vs. control to (i) quantify impact from a proof-of-concept, (ii) identify the performance drivers and (iii) most importantly to use these performance driver to predict results for a larger scale deployment, and to predict which locations and branches will perform the best in the next phase.
3:05-3:25pm • Room: Salon 3 & 4

Case Study: Hewlett Packard Enterprise
Operationalizing Analytics: 10 Key Process Areas for Embedding Predictive Analytics into Business Operations, Applications and Machines
Organizations who want to see measurable and sustainable business results from analytics must focus on embedding analytic processes and insights into day-to-day operations, which enable analytically driven decision making.
This session will cover the 10 key processes areas that must work together to support the seamless flow from initial analytic discovery to embedding predictive analytics into business operations, applications and machines.
Participants will receive a self-assessment survey designed to help determine their organization's maturity toward operationalizing analytics, along with recommendations on how to move forward at each stage.
[ Top of this page ] [ Agenda overview ]
3:25-3:55pm • Room: Salon 8 & 9
Exhibits & Afternoon Break
3:55-4:40pm • Room: Salon 3 & 4
Track 1: Crowdsourcing Predictive Analytics
Case Study: GE, Facebook and Walmart
What's possible at the cutting edge of predictive modeling
Kaggle is a community of almost 400K data scientists who have built almost 2MM machine learning models to participate in predictive modeling competitions. This talk will introduce machine learning competitions and will go over cutting edge applications, with case studies from companies like GE, Facebook and Walmart.
3:55-4:40pm • Room: Golden Gate A

Case Study: Lynda.com (a LinkedIn company)
Leveraging an Erroneous Treatment. Did We Wake Sleeping Dogs, Reactivate Engagement or Do Nothing at All?
Doing uplift modeling to identify hot and cold segments depends on a carefully designed experiment which is often not practical given business demands. In this case study, we examine the results of a fortuitous site error which sent "thanks for your payment" emails to all monthly subscribers rather than just those who had opted-in for the email. This resulted in higher than expected cancellations ("sleeping dogs") but also some reactivated subscribers ("persuadables"). You will learn the methods used and how the learnings were applied by Lynda.com (a LinkedIn company).
See also the full-day uplift modeling workshop on April 7
3:55-4:40pm • Room: Salon 5 & 6

Predictive Maintenance
Case Study: Microsoft
Predictive Analytics @work inside Microsoft: Revenue Modeling & Predictive Maintenance
In this talk, Microsoft machine learning practitioner Ivan Judson will present two predictive analytics models internal to the company: The first model is used to predict services issues that require human intervention in our cloud and datacenters to ensure a maximum customer experience while minimizing the cost of support, and the second model is used to predict annual world-wide and quarterly revenue from new work sold by consulting and comparing it to internal predictions by the CFO's office. Background, implementation history and details, and the performance of both models will be presented.
[ Top of this page ] [ Agenda overview ]
4:45-5:30pm • Room: Salon 3 & 4
Track1: Crowdsourcing Predictive Analytics
Case Study: City of Boston
Predicting Restaurant Violations via Yelp Reviews: Crowdsourcing for Social Good
Just like every major corporation today, nonprofits and governments have more data than ever before. And just like those corporations, they are eager to tap into the power of their data. But the social sector doesn't have the same resources to attract talent.
This talk covers how predictive can be applied to the challenges in the social sector. The first part is the big-picture context of the data for good movement and how to get involved. The second is an in-depth case study of the methods, which won DrivenData's recent machine learning competition and the results.
4:45–5:30pm • Room: Golden Gate A

Case Study: Facebook
Advanced Experimentation in Social Networks
At Facebook, we routinely work with very large datasets to drive product decisions; we run models to help us attribute and quantify marketing efforts to both sentiment and product usage (e.g., adoption, virality or time spent). Although many experiments are based on the simple concept of an A/B test, the pitfall is that you can't assume independence, since there are social connections between users in set A and those in set B. That is to say that interactions between users (sharing) would "contaminate" the control group. This dependence of one's response on other users is often referred to as "network effects". To address this issue, our network experiments takes into account the structure of the underlying network for the design (and perhaps analysis) of the experiment. In this talk, I will illustrate three recent user-facing products integrated into Facebook that the marketing analytics team analyzed, and the challenges we encountered as well as the insights we gained..
4:45–5:30pm • Room: Salon 5 & 6

Case Study: PayPal
eCommerce Churn - from Definition to Prediction to Reactivation
Consumer churn is a critical KPI for many organizations both subscription based and e-commerce. This presentation discusses a framework for quantitative understanding of churn, compares the performance various machine learning algorithms and lists out technical challenges and solutions. It begins with a set of simple probability distributions, explains the tasks performed during the exploratory data analysis phase and finally, compares the results from different machine learning algorithms: Random Forests, Gradient Boosting Machine, Support Vector Machines and Deep Learning, among others. It also details who the different consumers of the data product are and how it's presented to each persona.
[ Top of this page ] [ Agenda overview ]
5:30-7:00pm • Room: Salon 8 & 9
Networking Reception
Dinner with Strangers
Sign up in advance at the eZone, Booth 418
7:00-10:00pm • Room: Salon 5 & 6
Bay Area useR Group MeetingThe Bay Area useR Group (BARUG), the oldest R user group In the world, is the premiere venue in the San Francisco Bay Area for discussing the R language. Our mission is to share R knowledge and experience, and promote the use of R for statistical analysis and data science. Our monthly meetings which feature presentations on applying R to scientific, medical, financial, social and business applications attract R experts and beginners alike. BARUG meetups are a safe place to interact with others in the local R community, share knowledge and learn more about R. For more details about the agenda and the presentations please have a look at the BARUG meetup page for the event.
GLMNet Model: Prediction for the Oscars (Lightning Talk)
Keith R. Everett
Exploring R Packages to Estimate Conflict-Related Casualties in Syria
Megan Price, Executive Director: Human Rights Data Analysis Group
The ongoing conflict in Syria is extremely well documented. Through a combination of mainstream and social media, citizen journalists, and non-governmental organizations, many groups and individuals are carrying out the difficult and dangerous work of recording information about the violent conflict. But this information is unevenly distributed both over the time period of the conflict and the geographic regions of the country. The resulting missing data skew and bias any potential conclusions we may draw from observed patterns of violence. The class of statistical methods called multiple systems estimation (MSE) provide one way to estimate and account for this missing data. In this talk I will explore two R packages - dga and LCMCR - that implement Bayesian approaches to this class of methods. Code from each package will be demonstrated using preliminary analyses of data from the Syrian conflict.
Market Timing, Big Data and Machine Learning
Blair Hull, Ketchum Trading
There is a stigma against market timing. This stigma existed for good reasons, but the explosion of vast data sets and new analytical techniques has now made timing the market possible. Just as it was considered irresponsible to time the market over the last 30 years, it will be considered irresponsible NOT to time the market in the next 30 years.
Max Kuhn, Pfizer
7:00-10:00pm • Room: Golden Gate A
Bay Area SAS Users Group MeetingBay Area SAS Users Group (usually pronounced "Bay-Sas") is a local users group organized to further the interests of programmers and users of the SAS® Software in the San Francisco Bay Area, and to instruct members how to better use SAS® programming tools and user interfaces. Bay Area SAS Users Group also provides an arena for informing attendees and members of career opportunities.
A SAS® Macro to Compute Distance Correlation for Vectors
Thomas E. Billings, SAS Developer, MUFG Union Bank, N.A.
The Pearson correlation coefficient is well-known and widely used in analytics. However it has numerous weaknesses that constrain its usage, e.g., it is limited to univariate random variables and is a measure of linear dependence and not a general test of independence. Many alternatives to Pearson correlation have been proposed in recent years in the statistical literature.
This paper presents a SAS macro to compute distance correlation, an alternative correlation statistic that provides a test of independence and can work with vectors, matrices, or even text/non-numeric variables (so long as you can define a real-valued distance function between the variables).
Differentiate Yourself
Kirk Paul Lafler, Software Intelligence Corporation
Today's employment and employment marketplace is highly competitive. As a result, all SAS® professionals must learn how to differentiate themselves by acquiring the technical skills necessary to compete, and excel, in the global marketplace. This presentation illustrates how SAS professionals can begin enhancing their skills by accessing valuable and "free" SAS-related content.
With the aid of a web browser and the Internet, anyone can access published PDF "white" papers, Word documents, PowerPoint presentations, comprehensive student notes, instructor lesson plans, hands-on exercises, webinars, audios, videos, SAS Institute's comprehensive technical support website, and more to differentiate yourself and stand out from the competition.
Strategies for a Mixed Analytics Platform - SAS, Open Source, and Hadoop
Milan Lee, Technology Solutions Professional - Big Data Analytics, Microsoft
We are given more choices in data and analytics solutions than ever before. How do we make the best use of evolving SAS technologies while embracing the innovation from open source and Hadoop? In this session, we will explore the positioning of SAS, open source, and Hadoop within industry use cases, and discuss strategies to bring cohesiveness and efficiency to a mixed analytics environment.
For more information on the event, please go to www.basas.com
Conference Day 2: Tuesday, April 5, 2016
8:00-8:45am • Room: North Registration
Registration
8:00-8:45am • Room: Salon 8 & 9
Networking Breakfast
8:50-9:30am • Room: Golden Gate A
KEYNOTE
Case Study: Stitch Fix
Keys to Growing a World Class Data Science Team – Some Observations from Stitch Fix
Over the last couple of years, Stitch Fix has amassed one of the most impressive data science teams around. The team has grown from 5 to 50 people, collaborates with all areas of the business, and has a well respected data science blog plus several open source contributions.
As a member of this team since late 2014, and someone who has spent 15 years in the analytics space prior to that, I've often reflected on the "why" and the "how" behind this success of this team. What are the variables that may differentiate Stitch Fix when it comes to data science and what is the team doing differently? Can it easily be cloned or is Stitch Fix in a unique situation?
In this talk I will go through my observations since I joined Stitch Fix. Topics include, but are not limited to, the following:
- How the team grew. How to know when to hire?
- Maintaining high team morale and engagement.
- Achieving high productivity without traditional project management systems.
- What type of stuff are we working on? Is this unique to companies like Stitch Fix?
9:30-9:40am • Room: Golden Gate A
Plenary Session
Industry Trends: Highlights from the 2015 Data Miner Survey
In the spring of 2015, over a thousand analytic professionals from around the world participated in the 7th Rexer Analytics Data Miner Survey. In this PAW session, Karl Rexer will unveil the highlights of this year's survey results. Highlights will include:
- key algorithms
- challenges of Big Data Analytics, and steps being taken to overcome them
- trends in analytic computing environments & tools
- analytic project deployment
- job satisfaction
[ Top of this page ] [ Agenda overview ]
9:40-10:00am • Room: Golden Gate A
Diamond Sponsor Presentation
DataRobot: Better Prediction. Faster.
DataRobot is a platform for data scientists to build highly accurate predictive models, orders of magnitude faster than using traditional methods. Building an accurate predictive model generally requires searching through a near-infinite combination of data transformations, features, algorithms and tuning parameters. DataRobot simplifies model development by performing a large-scale parallel heuristic search for the best model or ensemble of models, based on the characteristics of the data and the prediction target. In this presentation, we will demonstrate the application using a real-world dataset of hospital discharge records of diabetic patients.
10:05-10:25am • Room: Salon 3 & 4

Open Source Lambda Architecture with Druid, Kafka, Samza, and Hadoop
The maturation and development of open source technologies has made it easier than ever for companies to derive insights from vast quantities of data. In this session, we will cover how to build a real-time analytics stack using Kafka, Samza, Hadoop, and Druid. This combination of technologies can power a robust data pipeline that supports real-time ingestion and flexible, low-latency queries.
10:05-10:50am • Room: Golden Gate A
Track 2: Retail Predictive Analytics
Case Study: SmarterHQ
The Revolution in Retail Customer Intelligence
In this new era of Big Data, retailers collect data in ever-increasing volume and variety. In the midst of Big Data, a revolution is taking place in how retailers gain insights about customers, whether they interact with the brand online, in stores, or both. This session will describe the transition from reporting to data-driven decisions using predictive analytics. Success requires collecting the right data, creating informative derived attributes, making this data accessible in a timely manner, and building predictive models. Examples, drawn from real-world retailers, will include shopping cart funnel management, shopping cart abandonment, marketing attribution, churn, and purchase propensity.
10:05-10:50am • Room: Salon 5 & 6
Track 3: Tracking Satisfaction Via Social Media
Case Studies: Capital One, Chase and Experian
How Well Do You Really Know Your Customer?
Data-driven segmentation can help you acquire new customers, but are you using it to deepen existing relationships? As organizations compete on the basis of customer experience, analyzing the customer lifecycle and ongoing journey becomes key. This case study focuses on how to measure the health of customer relationships utilizing a variety of data sources. Using the Consumer Financial Protection Bureau's complaint database, we track the performance of companies such as Capital One, Chase, and Experian. This session demonstrates how predictive analytics can uncover customer complaints in social media, how to track emerging trends, and how to improve negative customer experiences.
10:30-10:50am • Room: Salon 3 & 4

Hadoop for Predictive Analytics - A Data Scientist's Secret Weapon Against Malware Threats
The IT environment is rapidly changing: new technology stacks emerge every year that serves billions of people worldwide. However, many of these new technologies have not been thoroughly tested, and as a result, malware writers have targeted them. How can you quickly and effectively distinguish a network intrusion attempt from an expected and authorized event? A great approach for getting in front of those attacks involves the use of big data technologies for predictive analytics. By analyzing all your network event data with Apache Hadoop and Apache Spark, you can build models that identify normal behavior as well.
[ Top of this page ] [ Agenda overview ]
10:50-11:20am • Room: Salon 8 & 9
Exhibits & Morning Coffee Break
11:20–11:40am • Room: Salon 3 & 4
Track 1: Self-Serve Prediction; Network Security
Case Study: Incapsula
Predicting the Extent and Cost of Online Attacks to Help Sell Security Software
Incapsula turned a predictive model into an online sales tool, resulting in significant increases in overall sales lead quality and volume. A predictive model was developed in order to calculate the probability and associated downtime cost of distributed denial-of-service (DDoS) attacks a company will experience. This model was then transformed into a web-based application, which enables potential Incapsula software customers (companies) to answer a few simple questions and receive a projection of their level of risk of an attack.
11:20am–12:05pm • Room: Golden Gate A

Case Study: Workday
Time-Series Feature Engineering Done Right
We are building a data platform to allow our ML engineers to rapidly create predictive applications.
The biggest challenge we\'ve had so far has been with validation. It turns out that when you have historical data where the events are correlated, it's easy to make mistakes with validation and assume that your algorithms are performing better than they really are.
In this talk, we'll present a case for using temporal validation over traditional approaches, such as cross-validation, when applying machine learning on historical data sets.
You will not need significant experience Machine Learning to understand this talk.
11:20am–12:05pm • Room: Salon 5 & 6

Q&A: Ask Karl and Steven Anything (about Best Practices - for Financial Services and Beyond)
Beyond the Arc CEO Steven Ramirez, along with Rexer Analytics president Karl Rexer, field questions from an audience of predictive analytics practitioners about their work, best practices, and other tips and pointers.
11:45am–12:05pm • Room: Salon 3 & 4
Track 1: Employee Theft Detection
Case Study: Major Fashion and Apparel Retailer
Caught in The Act: Loss Prevention Rules Firing & Alerts
The amount of employee theft is always a huge problem and is weighing heavy on dishonest employees who steal approximately 5.4 times the amount stolen by shoplifters. The National Retail Security Survey estimates that over $44.25 Billion dollars annually is stolen from retailers. Companies are placing a renewed emphasis on managing shrinkage to help improve profitability.
By using sophisticated analytics, Companies can make better loss prevention decisions. A small reduction in shrinkage can have significant impact on the bottom line. Companies that implement advanced analytics into their company and supply chain pipeline are seeing a significant decline in theft.
[ Top of this page ] [ Agenda overview ]
12:00–1:15pm • Room: Salon 8 & 9
Lunch in the Exhibit Hall
2:15–3:00pm • Room: Golden Gate A
Special Plenary Session
Doing Space-Age Analytics with Our Hunter-Gatherer Brains
Predictive Analytics is so powerful and so useful – everywhere – we are astonished that its widespread adoption has taken so long. Its modest risk and phenomenal return should lead rational actors to cooperatively pool technical and domain expertise to tweak production processes to the benefit of all. And yet, most early projects fail to be implemented – felled by fear, pride, and ignorance.
But we can anticipate those foes! Recall that success requires solving three serious challenges: 1) Convincing experts that their ways can be improved, 2) Discovering new breakthroughs, and 3) Getting front-line users to completely change the way they work. No wonder there is resistance at every stage!
John argues that it's helpful to have a mental model of the human brain as not optimized for success in our modern life of safety and abundance, but for survival within a small tribal society. And that with this model we can better anticipate – and escape - the traps that we idealistic techno-nerds tend to blunder into as we try to bring life-changing fire into the tribal circle.
Lightning Round
2:15–3:00pm • Room: Golden Gate A
Expert Panel
Data Prep: Overcoming the Bottleneck and Nailing It
Machine learning reigns supreme - but, alas, you first need examples from which to learn. The vast majority of hands-on time for most predictive analytics initiatives is spent preparing the data. Estimates generally hover around 80%. To realize the great potential of predictive modeling, you must form training data that consists of one row per training example, each consisting of a list of predictor (independent) variables, plus the target (dependent) variable that you plan to model/predict. The data prep is always a specialized task, which can only at best be partially automated and is replete with elusive pitfalls and unexpected delays. This expert panel will address:
- Tricks of the trade for data prep
- Tools, methods, and techniques
- Anecdotal successes and hard lessons learned
Moderator:
Panelists:
3:00–3:30pm • Room: Salon 8 & 9
Exhibits & Afternoon Break
3:30–4:15pm • Room: Salon 3 & 4
Track 1: Social Data for Predictive
Case Study: Cars.com
Predicting Consumer Review Engagement and Sentiment Using only Readily Available Social and Demographic Data
One of the most universal, and unfortunately often dreaded, consumer experiences is with the automotive dealership. The vast majority of these experiences are actually positive and dealers need to ensure that these positive experiences result in public, online dealership reviews. This case study addresses the issue of whether it is possible to create actionable analytics based on currently available social and demographic data to determine which consumers are likely to generate a review and therefore positively impact the dealer's online reputation. This predicative analysis would actually make it possible to counteract the impact of disproportionate negative voices in the consumer social network – helping automotive dealerships – and likely other online reputation dependent businesses – create a more balanced and informative market view.
3:30–4:15pm • Room: Golden Gate A
Track 2: Open Source Tools
Case Study: Facebook
Predictive Analytics on the Command Line
Python and R are popular, powerful tools for predictive analytics. Today, it has never been easier to build integrated Python and R scripts and rapidly develop analyses on the command line using tools such as csvkit, drake, Rio, pandashells, and skll. Come learn how Facebook\'s infrastructure data scientists use these tools to inform hardware acquisition and maintenance decisions and data center operations decisions.
3:30–4:15pm • Room: Salon 5 & 6
Track 3: Insurance
Case Study: The
Co-operators
Developing an Analytics Practice and a Science Culture in Insurance
This is a proven and practical approach to building a successful research and analytics program. Although the examples will relate to the P&C and life insurance industry, most of the contents apply to other industries as well. This talk will include how to get started even without a budget, and achieve benefits early. There will be examples of high value / low risk predictive analytics applications, with measurable results. Learn also how to make the business case for analytics, build your team with the right mix of profiles, and create a culture of science and innovation in your organization.
[ Top of this page ] [ Agenda overview ]
4:15-5:00pm • Room: Salon 3 & 4
Track 1: B2B Sales; Energy
Case Study: Omaha Public Power District
Predictive Sales Targeting in the Energy Industry
Our local power company's economic development department sought a tool to help them identify small-to-medium companies they should target for sales and marketing activities. To that end, our predictive model took into account economic numbers, taxes, graduation rates, employment rates, population growth, power/water/Internet prices, NAIC codes, manufacturing/production types, and growth indexes across 7 disparate databases. We scored over 100,000 companies based on the county through which sales staff were traveling and presented the information in a dashboard that could be explored by the team prior to travel.
4:15-5:00pm • Room: Golden Gate A
Track 2: Healthcare Analytics
Case Study: Sutter Health
Overcoming Big Data Bottlenecks in Healthcare: A Predictive Modeling Case Study
There are big bottlenecks in the use of big data in healthcare. We face bottlenecks in access to and analysis of data in near real-time, and in the integration of analytics into every day care practice.
To highlight these challenges, we will explore transitions in care. Health systems are challenged today to reduce 30-day readmissions. Proactively identifying patients with the greatest risk of readmission and delivering personalized care is the backbone of population health management (PHM).
Implementing PHM in transitional care settings is challenging because of: 1) Data interoperability and other bottlenecks 2) complex workflows designed for reactive rather than proactive processes; and 3) difficulty in integrating them into clinical workflows
We present a use case demonstrating a practical, real-world solution to these challenges.
Three audience takeaways from presentation:
- Learn about the big data bottlenecks in healthcare
- Learn how Sutter Health is using its E.H.R. data in a readmission risk predictive model;
- See how those predictive models are integrated into clinical operations in improving care
4:15-5:00pm • Room: Salon 5 & 6
Track 3: Optimizing Discount Pricing
Case Study: Paychex
Maximize Value and Retention With Predictive Analytics In Discounting
Today's businesses face a challenge: building discount structures that allow them to attract and retain customers, without diminishing the value of the products and services they provide. Join us for an in-depth look into a predictive modeling use case on discounting to see how a combination of models can help answer the questions, what is the right discount for a particular client, and how sensitive are clients to the changes and expiration of discounts.
[ Top of this page ] [ Agenda overview ]